Story
Why does one patient respond to a cancer treatment, while the other does not? Researchers in the lab of Oncode Investigator Lodewyk Wessels (Netherlands Cancer Institute) shed light on that question by developing computational models based on multiple data sources. Their ultimate goal: modeling an entire cell to predict drug response.
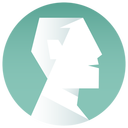
Wessels Group
Lodewyk Wessels - Netherlands Cancer Institute
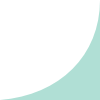
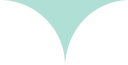
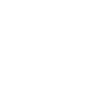
Constructing the ultimate model
How can a model aid us to understand or even predict drug response in a cancer patient? “That is our main question”, says Oncode Investigator Lodewyk Wessels. “We profile tumours and quantify treatment response. We try to understand that relationship through computational modeling. By collecting large datasets, constructing and training these models, we gradually work towards an in silico model of an entire cell. That is our long-term perspective.”
Wessels started his scientific career at the University of Pretoria in South-Africa. As a graduated engineer, he worked towards his PhD degree in the field of intelligent control at the Oregon Graduate Institute of Science and Technology in Portland, Oregon. That path brought him to the TU Delft for a postdoc in the field of intelligent diagnostics for early detection of disease. “This was around the time when the human genome was being sequenced”, says Wessels. “Lots of laboratories were performing gene expression profiling but didn’t have the expertise to analyze the data. With our background in machine learning, we were able to obtain results.”

Understanding the wiring
Their success in computational biology led to multiple successful scientific co-operations, amongst others, with the Netherlands Cancer Institute. There, in 2006, he became a group leader in the field of computational cancer biology. Since then, Wessels extended his research group at the NKI to its current size of around twenty people, many of them having shared appointments with other research groups. However, all of them contribute in one way or another to further our understanding of therapy response. Both on a larger, data-driven scale using statistical approaches, as well as with mechanistic models of selected pathways.
To understand how the cancer genome translates into the complex biological behavior of cancer cells, we have to understand which parts of the DNA encode this behavior. Wessels and his team develop statistical models to analyze this genetic data. “Some genes are so frequently mutated that they surpass statistical expectation”, says Wessels. “Using algorithms, we can extract that from the data. Our models predict when a gene exceeds the background noise, providing evidence that that gene is important in cancer.” The second step is understanding how all individual effects of these mutations can be combined. To achieve this, they work on understanding the connections between individual proteins. “By mapping pathways in our models, we can understand cellular decision making, and how these interactions affect drug response”, says Wessels. “If we understand how pathways are wired, we might understand why certain therapeutic combinations work, and others do not.”
Some genes are so frequently mutated that they surpass statistical expectation

Confounders in synergy
But before that can happen, there still are a few hurdles Wessels and his colleagues must overcome. One of them is to study the synergy between mutations involved in cancer by applying statistical algorithms on big datasets. Joris van de Haar, a medical doctor and PhD student in the Wessels and Voest labs, studies confounding variables in these analyses, such as mutational load and synergy. “The current reality is that patients are often treated based on a single mutation in their tumor”, says Van de Haar. “Most of the time, that’s just too simplistic.”
One of Van de Haar’s findings is that most of the statistical patterns used to detect synergies between mutations are frankly misleading. “They arise through a broad set of confounding factors, rather than through synergy”, says Van de Haar. In order to really understand how combinations of mutations drive cancer, he and his colleagues are developing models to overcome this. Still, he remains cautious about the use of genetic data in the clinic before we know how to interpret these. “Before we start using the knowledge of interactions between mutations in the clinic, it is important to know that there often are other possible explanations.”

The sum of its parts
Synergistic interactions are not only applicable to mutations. Scientists, led by fellow Oncode Investigator René Bernards, discovered that in administering anti-cancer drugs, the whole is more than the sum of its parts too. Using very low doses of multiple drugs, they were able to completely inhibit the growth of an extremely drug resistant form of lung cancer by inhibiting multiple proteins in a single pathway. To unravel the mechanisms behind this finding, researchers from the Wessels group are now collaborating with the Bernards lab.
“For me, this is where the fun starts”, says Bram Thijssen, postdoc in the Wessels group. In his work, he is standing with one foot in statistics and with the other one in computer science. “We use genetic data from patients as well as from single cells. We compare our pathway model with the data to see if it fits.” The main question that keeps Thijssen busy on a daily basis is how to make his models fit as well as possible, while making reliable predictions. “A model is never completely right, so one always has to make corrections. An important part of my job is to make our models as robust as possible by calculating how big that correction has to be.”

Model refinement
Eventually, the research of Wessels and his colleagues might lead to models that have specific clinical implications, such as predicting drug response. “The use of our models in daily clinical practice is a great future perspective and would be very rewarding”, says Thijssen. “In time, doctors might use them as an extra tool to help them decide which treatment has the best chance of success.” The models of the Wessels group might also be a steppingstone to developing more complex models predicting the decisions made in cancer cells, based on its sensitivity for certain drugs. Wessels says: “The Oncode base fund is of great importance to our lab, since it gives us total freedom in developing our models. With these models, we try to build the foundation for future clinically applicable research.”
But despite all the current research, that vision will probably still take years of further refinement. “The clinical implementation of computational models is still no reality yet”, says Van de Haar. “But it fits in a vision. Eventually, we will use artificial intelligence and computer modeling to understand treatment effects in individual patients.” Van de Haar thinks that the cooperative spirit in the Wessels lab helps to fulfill that vision. “This is one of the cornerstones of the lab. We all have different backgrounds – biology, computer science, mathematics, medicine – but learn to speak each other’s language. In our lab, all these worlds collide. That makes it a place where great things can come into existence.”
Credits: interview by Koen Scheerders; photography by Marloes Verweij, Laloes Fotografie